Understanding Science
If we don't take it upon ourselves to understand science, others will do it for us. And their motives and incentives may not be in our best interest.
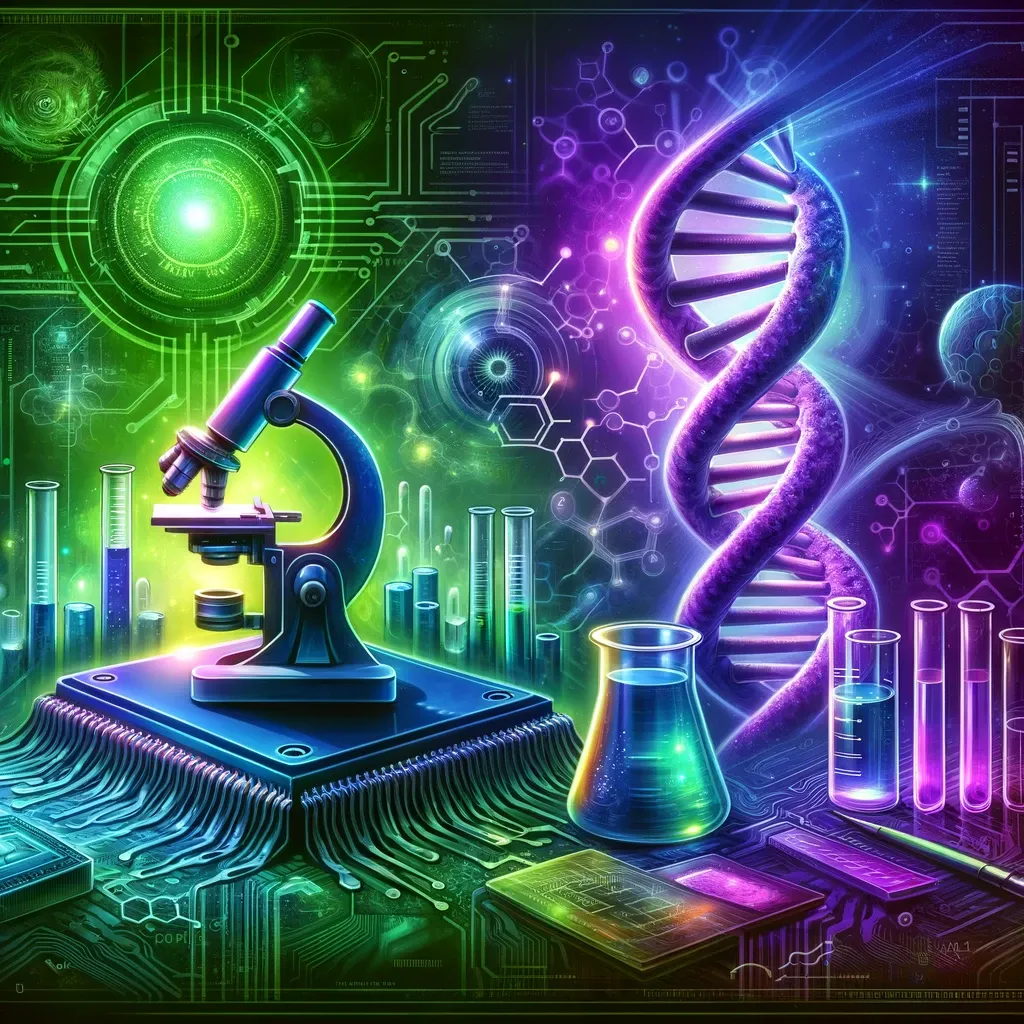
A deeper understanding of science should be required of everyone. And we should trust carefully. If we leave it to others, we are at the mercy of their motives and incentives.
The most important thing about science is that we should appreciate it. The process of scientific discovery has given us much of what we enjoy in the modern world – the lifespan and quality of life; the conquest (mainly) over disease and malady.
It's a quest for the truth.
Science, like all tools, is something that can be used for good or ill. Generally it has been the engine of progress. It should also inspire awe, which Bill Bryson’s A Short History of Nearly Everything does well.
Like democracy, the scientific process isn't perfect, but it's the best one we've got. I fear we take both for granted. There are 2 general categories of where science can go wrong. The first is within the process itself and the second is going from scientific finding to policy that improves our lives.
Part 1 – Where Science Can Go Wrong
Stuart Richie's Science Fictions tells us more about where the scientific process can go awry. For that, you need an understanding of what the replication crisis is and why it is such a big problem.
The first thing to understand when considering some kind of scientific finding: just how unusual is your result? Let's suppose you have a petri dish with some cells and apply some new nutrients. If you see 20% cell growth you will need some way of divining exactly what that means. Is that more than normal? Less? By how much? And is that difference meaningful? You can think of this as a base rate.
There's a statistical tool to help you answer this: the p-value. Briefly, it's meant to capture how likely your result would be observed under the baseline case that your intervention had no true effect. Many traditional publications set this value at 5%. (A side note: someone has coded up a very amusing p-value-hacking site supported by AI.)
The next thing to understand is the concept of replication: the process by which an independent group tries to reproduce the findings in a specific scientific paper. This is not glamorous work. No one will get tenure off of it. There's no originality and very few scientists see their life's work as replicating the findings of other labs. Remember from p-value that there is still some chance of observing these results even if the treatment actually has no effect.
In theory, if you have a p-value of 5% for a field, then you would expect around 5% of papers to fail to replicate. For all processes, it's good to have some expectation of base rate to understand how surprising some finding would be. In the case of psychology, 60-80% of papers fail to replicate. In plain language, most of the “scientific findings” are not true. Something has gone terribly wrong.
Why does this happen? Science Fictions gives us 4 major reasons: Fraud, Bias, Negligence, and Hype.
- Fraud: lying about some aspect of the study or fabricating data. This contributes but isn't everything. When you see a paper retracted it means that an institution has discovered or been alerted to this type of fraud.
- Bias: we're much more likely to publish something exciting meaning that people are motivated to find exciting results. This lends itself to a publication bias and the desk-drawer problem. XKCD has an excellent illustration of p-value hacking here.
- Negligence: the category where a skilled, diligent scientist doesn't make that same mistake. Apparently a series of errors in biology papers happened because of mis-use of microsoft excel (as many as one in five genetics papers). This category also includes not calculating the p-value correctly or not using all the data correctly in a spreadsheet.
- Hype: the more exciting the field, the more there's a rush to publish and the more “desk drawers” would exist. This is a magnifier on bias and I think a big issue affecting machine learning at present.
One mitigation against some of these issues is to require companies to pre-register trials. Science Fictions shows what happens when you do:
Note the large shift in distribution and how we finally see a red result.
Another sign that something is amiss: you see a negative correlation between experiment size and results. A natural set of experiments should have a “pyramid” of results. The largest studies should cluster tightly around a mean, and the smaller ones should have a “wide” base of higher and lower results around that mean. This is just the nature of statistical power. However, if there's publication bias, the lower left part of the pyramid would be obscured, leading to this negative correlation.
So science isn't perfect. But it becomes a real issue when it's misrepresented to be used against the general interest.
Part 2 – Where It Can Be Used Against Us
The first well-known incident where industry conspired to keep scientific understanding from becoming law concerned leaded gasoline. Initially gasoline and car motors had a big problem: the engines would knock and cause some damage to cars. A solution was found: by adding lead to gasoline. It took until 2021 for it to be phased out of cars despite being banned from new cars in 1975. In the meantime, many people in the United States grew up with developmental deficiencies because of environmental lead. Oddly, this story is not covered in an otherwise informative book Merchants of Doubt.
Merchants of Doubt outlines a similar pattern of doubt and denial to delay sensible regulation. That book includes the public-information-debate histories of tobacco, acid rain, ozone holes and global warming.
The biggest criticism of Merchants would be that these are all issues that, in hindsight, obviously should have been regulated sooner and more strictly. I’m more curious to know a bit about the false positives – where caution or delay was actually appropriate or that we rushed to suppress something faster than we should have. I can think of several contemporary examples (think of divergent regulation between the EU and the US and you’ll get a sense of some of the contemporary issues).
It’s still likely the simplistic story of industry blocking sensible regulation is true, but this book is one-sided. That acknowledged, the book does clearly catalog a litany of bad behavior but non-truth seeking parties to muddy a debate.
Some general advice based on this book:
- Don’t trust a scientist just because they’re a scientist – for example a physicist opining on biology, or a rocket expert opining on the environment
- Be aware of the use of doubt (hence the title) to mislead you on where the scientific understanding lies. Examples from the tobacco battles include (and apologies for the quote at length, but it best illustrates this concept):
Why? Why do cancer rates vary greatly between cities even when smoking rates are similar? Do other environmental changes, such as increased air pollution, correlate with lung cancer? Why is the recent rise in lung cancer greatest in men, even though the rise in cigarette use was greatest in women? If smoking causes lung cancer, why aren’t cancers of the lips, tongue, or throat on the rise? Why does Britain have a lung cancer rate four times higher than the United States? Does climate affect cancer? Do the casings placed on American cigarettes (but not British ones) somehow serve as an antidote to the deleterious effect of tobacco? How much is the increase in cancer simply due to longer life expectancy and improved accuracy in diagnosis? None of the questions was illegitimate, but they were all disingenuous, because the answers were known: Cancer rates vary between cities and countries because smoking is not the only cause of cancer. The greater rise in cancer in men is the result of latency—lung cancer appears ten, twenty, or thirty years after a person begins to smoke—so women, who had only recently begun to smoke heavily, would get cancer in due course (which they did). Improved diagnosis explained some of the observed increase, but not all: lung cancer was an exceptionally rare disease before the invention of the mass-marketed cigarette. And so on.
- Keep in mind that industry is often much better funded than general research – and while trying to pay attention to funding sources you may not get a full and clear picture.
- Be wary of strong conclusions based on weak data. Sometimes this is done accidentally (see part 2) but it can also be done deliberately or in bad faith. Merchants identifies several scientists that did this for the sake of ideology – that of freedom or liberty over regulation.
Merchants leaves us with this parting thought:
So it comes to this: we must trust our scientific experts on matters of science, because there isn’t a workable alternative. [But o]ur trust needs to be circumscribed, and focused. It needs to be very particular.
What’s the best way to implement this guidance? I suggest investing some time in learning how science happens and thinking systematically about how the information is coming to you. Think deeply about what sources of information, publication and person, that you trust. And when faced with a new idea, try to integrate it with your mental model of the world–ask yourself if that result and interpretation makes sense.