Economics and AI -- Book Review: Power and Prediction
Book reviews for 1) Power and Prediction and 2) Prediction Machines. The same authors are economists who tell us about how AI may develop.
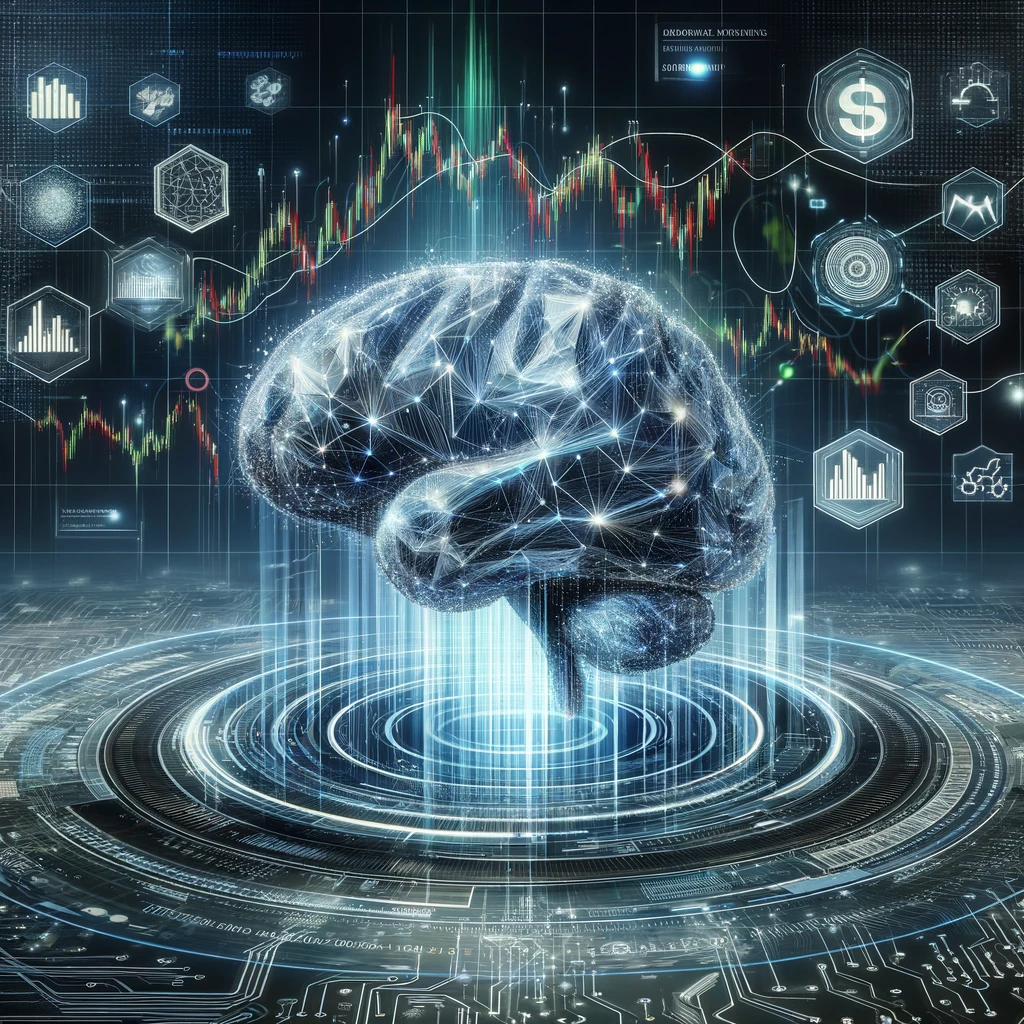
The book Power and Prediction by Agrawal, Gans, and Goldfarb examines how businesses can best leverage AI. It argues that rather than focus on point solutions that improve individual decisions, firms should pursue system solutions that fundamentally redesign interconnected decisions. In order to understand the full potential of AI, thinking in systems is where the greatest value will lie.
The authors describe three types of AI solutions. Point solutions involve an AI prediction that enhances a single decision. The industrial revolution analogue: replacing a steam engine with an electric engine in the exact same place of the factory floor. Application solutions allow AI to enable new decisions or change how they are made, but still independently. To continue the analogy: distributing power to individual motors in a factor rather than from a central shaft. System solutions, the most valuable but difficult to implement, require redesigning multiple decisions that depend on each other. For example, a modular factory like what Henry Ford designed.
Their previous book, Prediction Machines, made the point of separating predictions from judgment. Before AI this distinction didn’t mean too much in practice. Now it matters a lot: a prediction could be the chance someone has a disease, but the judgment is what to do with that information.
Many business rules exist to reduce uncertainty. The book discusses how AI can turn these rules into data-driven decisions, unlocking value. But uncertainty leads organizations to establish interdependent rules and processes that resist change. The authors use the example of modern airports full of rules managing uncertainty – if you knew all the airport delays with perfect certainty you would not aim to spend time in the lounge. By contrast, think of a private airport’s lounge–positively spartan.
Education is another system anchored by rules, like age-based curricula, rather than personalized decisions. Dropping an AI point solution into the current model would really be a waste of the potential of AI. Instead, making it learner and task-based would be closer to a system solution. Even more powerfully, the AI can be designed to inspire curiosity and other longer term traits, which are almost completely missing from the current, standardized teaching. AI could incorporate the latest in research about learning styles, optimized repetition for a personal Ebbinghaus’s forgetting curve, and so on.
As economists, the authors emphasize evaluating AI based on new value creation versus cost savings. For example, AI can generate better hypotheses, accelerating experimentation and innovation. This contrasts with a short-term, task-automation mindset. The truly disruptive applications of AI will rethink entire value chains and not automate a specific task within the existing one in a point solution.
Power and Prediction also examines competitive dynamics. First movers benefit from feedback loops as their AI system learns. But others can catch up unless the learner secures a data advantage. For instance, personalized recommendations improve with more user data. Reinforcing feedback loops sustain first-mover advantage.
The insurance industry provides another example of reimagining systems beyond automation. Rather than just better pricing risk, insurers could use AI to advise customers on mitigating hazards, transforming to risk prevention partners. While insurance in the case of a rare catastrophe would be important, advanced AI could also help individuals reduce their risks and get paid to do so. This already exists in some form with the safe driver apps built upon a lot of individual driving data.
The book argues that instead of focusing narrowly on point applications, businesses should pursue integrated solutions that rethink entire systems in light of AI capabilities. This requires judgment around where predictions should reside and thoughtfully reallocating control. Power and Prediction makes the case that firms who reimagine their systems will generate the most value from AI's predictive breakthroughs. When predictions scale as the result of AI, scarcity and thus economic power, will likely move elsewhere in the value chain. For every industry in question, it’s not clear where scarcity will reside.
Link to a podcast interview with Avi Goldfarb.
Notable Quotes:
“We define power as economic power. You have power if what you own or control is scarce, relative to demand.”
“Those resistant to change often currently hold power (that’s why they resist) and therefore may be quite effective at preventing system-level change. That creates the context for disruption.”
“Feedback loops can have significant implications for system design. In order for an AI to learn, it must have access to the outcome data.”